AI Predictive Maintenance: A Leader's Guide to Cost Savings
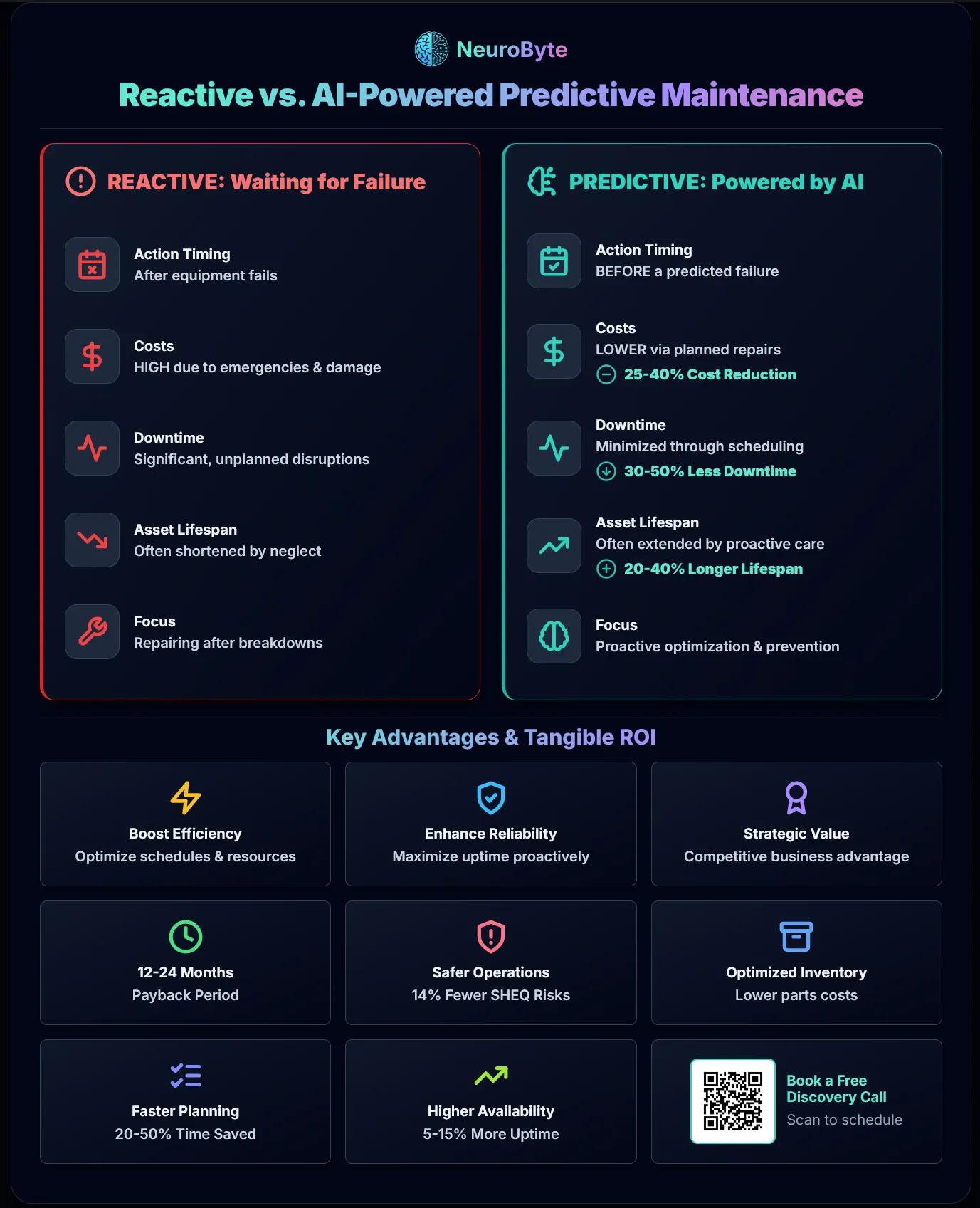
From Reactive to Predictive: A Leader’s Guide to Slashing Maintenance Costs with AI
Executive Summary
AI-powered Predictive Maintenance (PdM) enables organizations to anticipate equipment failures, reduce unplanned downtime, and optimize maintenance costs. This guide provides business leaders with a clear roadmap to move from reactive to proactive maintenance, quantifies the ROI, and highlights real-world results. Learn how to leverage your operational data for strategic advantage, avoid common pitfalls, and drive measurable business value through AI-driven maintenance.
Unplanned equipment downtime isn’t just an operational headache; it’s a major drain on profitability. Reactive maintenance—fixing things after they break—causes spiraling costs, missed production targets, and safety hazards. What if you could predict failures before they happen?
Artificial Intelligence (AI) is transforming maintenance strategies. AI-powered Predictive Maintenance (PdM) uses data from your machinery and operations to anticipate issues. This allows you to schedule maintenance proactively, optimize resource allocation, and turn your maintenance function from a cost center into a strategic advantage.
This guide is for business leaders and executives. We’ll cut through the technical jargon to clarify:
- The True Cost of Reactive Maintenance: Uncovering hidden expenses.
- The Predictive Maintenance Advantage: How AI changes the game.
- Making the Business Case: Calculating the Return on Investment (ROI) of AI-powered PdM.
- Your Implementation Roadmap: A step-by-step approach to getting started.
- Real-World Results: Seeing the impact through examples.
- Overcoming Hurdles: Navigating common implementation challenges.
- Finding the Right Partner: Key considerations for success.
Ready to shift from reactive firefighting to proactive optimization? Let’s dive in.
The Steep Price of Waiting for Failure
Reactive maintenance might seem simpler initially, but the cumulative costs are staggering. It’s more than just the repair bill; the ripple effects impact your entire operation:
- Lost Production: Every minute of unplanned downtime means your assets aren’t generating revenue.
- Expensive Emergency Repairs: Premium charges for expedited parts, overtime labor, and rushed shipping add up fast.
- Reduced Asset Lifespan: Running equipment until failure often causes excessive damage, shortening its usable life.
- Inventory Issues: Production halts can cause stockouts or force you to maintain higher safety stocks, tying up capital.
- Safety Risks: Equipment failure can create hazardous conditions for employees.
- Reputational Damage: Failing to meet delivery schedules or quality standards due to downtime erodes customer trust.
The Hidden Costs of Reactive Maintenance
Direct Costs
- Emergency Repairs
- Overtime Labor
- Expedited Parts Shipping
Indirect Costs
- Lost Production & Revenue
- Reduced Asset Lifespan
- Safety Hazards
- Inventory Issues
- Reputational Damage
Think Beyond the Repair Bill
Unplanned downtime is more than just a repair cost—it includes lost production, expedited repairs, safety incidents, and reputational damage.
Unplanned downtime can cost industries on average $260,000 an hour for critical failures.
(Source: Aberdeen Group, 2016)The Predictive Maintenance Advantage: Seeing the Future
Predictive Maintenance (PdM) uses data analysis and machine learning algorithms (AI techniques that learn from data) to detect subtle patterns and anomalies signaling potential failure. Instead of relying on fixed schedules (preventive maintenance) or waiting for breakdowns (reactive maintenance), PdM enables you to:
- Predict Failures: Identify potential issues days, weeks, or months in advance.
- Optimize Maintenance Schedules: Perform maintenance only when needed, based on actual equipment condition.
- Reduce Downtime: Schedule repairs during planned outages to minimize disruption.
- Extend Asset Life: Address minor issues before they become major failures.
- Improve Safety: Proactively identify and mitigate potential equipment hazards.
- Lower Costs: Cut emergency repair expenses, optimize spare parts inventory, and improve labor efficiency.
Key PdM Benefits
- Predict Failures: Identify potential issues days, weeks, or months in advance.
- Optimize Maintenance Schedules: Perform maintenance only when needed based on actual condition. This can lead to a 20-50% reduction in planning time. (Source: Deloitte, 2017, via Infraspeak)
- Reduce Downtime: Schedule repairs during planned outages to minimize disruption.
- Extend Asset Life: Address minor issues before they become major failures.
- Improve Safety: Proactively identify and mitigate potential equipment hazards.
- Lower Costs: Cut emergency repair expenses, optimize inventory, and improve labor efficiency.
Predictive Maintenance Pros & Cons
Pros
- Reduced Downtime: Proactive scheduling minimizes disruptions.
- Lower Costs: Prevents major failures, optimizes labor & parts.
- Extended Asset Life: Addresses issues before significant damage.
- Improved Safety: Identifies potential hazards early.
- Data-Driven Decisions: Optimizes maintenance schedules based on need.
Cons
- Higher Initial Investment: Requires sensors, software, expertise.
- Data Requirements: Needs quality data for effective modeling.
- Complexity: Implementation involves data integration and ML skills.
- Change Management: Requires shift in team workflows & mindset.
- Potential for False Positives/Negatives: Models aren't always perfect.
The PdM Edge
PdM shifts maintenance from a reactive necessity to a proactive, data-driven strategy. By anticipating failures, businesses can significantly reduce operational disruptions, lower maintenance costs, and extend the life of their valuable assets.
How does it work (in simple terms)?
PdM Operational Flow
- Yes: Proceed to Failure Prediction & Correlation.
- No: Continue Monitoring with Established Baseline.
Making the Business Case: Quantifying the ROI
Implementing AI-powered PdM requires investment, but the returns can be substantial. Building a strong business case means quantifying the potential savings and benefits:
Key ROI Drivers:
Maintenance Cost Reduction
Potential reduction in maintenance costs compared to reactive strategies. (Source: McKinsey, PWC)
Downtime Reduction
Potential decrease in unplanned downtime through proactive repairs. (Source: Deloitte, Infraspeak)
Asset Lifespan Extension
Potential increase in equipment lifespan by addressing issues early. (Source: McKinsey, PWC)
These figures represent commonly cited industry benchmarks for PdM effectiveness. For further details and consolidated sources, refer to industry reports such as those from Infraspeak, which compile findings from firms like McKinsey and PWC .
- Increased Asset Availability: PdM can lead to a 5-15% increase in asset availability (Source: McKinsey, 2018, via Infraspeak) .
- Improved Safety & Compliance: Avoid costly accidents, potential regulatory fines, and reduce safety, health, environment, and quality (SHEQ) risks by up to 14% (Source: PWC, 2018, via Infraspeak) .
- Optimized Inventory: Reduce the need for large, costly spare parts inventories.
ROI is often realized within 12-24 months.
Simple ROI Framework:
- Establish Baseline: Calculate your current annual reactive maintenance costs (downtime losses, repair costs, etc.).
- Estimate PdM Savings: Project potential reductions in each cost category using industry benchmarks and pilot results (e.g., 25% lower repair costs, 40% less downtime).
- Calculate Implementation Costs: Include costs for sensors (if needed), software, data infrastructure, training, and consulting.
- Determine Payback Period & ROI: Calculate how quickly savings recoup the initial investment and the overall return percentage.
Illustrative ROI Calculation
Inputs
Baseline Annual Cost
e.g., $500k / year
Estimated PdM Investment
e.g., $100k
Projected Outcomes
Projected Annual Savings
e.g., $150k / year (30%)
Estimated Payback Period
~8 months
Estimated ROI (Year 1)
50% (Year 1)
Note: These figures are illustrative. Actual ROI depends on specific operational context and implementation details.
Data is Key
PdM success hinges on having the right data. This often includes sensor readings (vibration, temperature, acoustics), operational logs, maintenance records (often from a CMMS - Computerized Maintenance Management System), and asset details. Don’t worry if your data isn’t perfect; a data assessment is a vital first step.
CMMS (Computerized Maintenance Management System)
Software used by organizations to manage maintenance activities, track work orders, schedule tasks, and store asset information. Integrating PdM insights into a CMMS streamlines workflows.
Your AI-Powered PdM Implementation Roadmap
Transitioning to predictive maintenance is a strategic initiative, not just a tech upgrade. A phased approach ensures buy-in, manages risk, and delivers value step-by-step.
Assessment & Strategy
Action: Pinpoint your most critical assets where failure causes significant impact.
Goal: Set measurable business objectives (e.g., reduce unplanned downtime on Asset X by 30% within 12 months).
Focus: Audit your existing data sources (sensors, logs, CMMS), assess data quality and accessibility, and evaluate potential technology platforms.
Pilot Project
Action: Choose 1-2 high-impact critical assets for an initial pilot.
Focus: Implement necessary sensors (if needed) and establish data pipelines.
Goal: Build baseline AI models to detect anomalies and predict failures for the pilot assets. Validate predictions with your maintenance team's expertise.
Data Preparation & Integration
Action: Cleanse, transform, and unify historical and real-time data from various sources (sensors, CMMS, ERP, SCADA).
Goal: Create a reliable, integrated dataset suitable for training robust AI models.
Focus: Address data gaps, inconsistencies, and formatting issues.
Model Development & Deployment
Action: Select appropriate machine learning algorithms (e.g., for time-series analysis, anomaly detection, classification).
Focus: Train and rigorously test the models using the prepared data. Tune model parameters for optimal accuracy and reliability.
Goal: Deploy the validated models into a production environment where they can process live data.
Integration & Change Management
Action: Integrate PdM alerts and insights directly into existing maintenance workflows and systems (e.g., CMMS work orders, dashboards).
Focus: Train maintenance planners and technicians to interpret predictions and act on insights.
Goal: Foster collaboration between operations, maintenance, and data teams. Manage the transition from traditional to predictive maintenance practices.
Monitoring & Continuous Improvement
Action: Continuously track Key Performance Indicators (KPIs) related to maintenance costs, downtime, and asset health.
Focus: Monitor model performance and retrain models periodically with new data to maintain accuracy.
Goal: Gather feedback, refine processes, and plan the scaling of the PdM program to additional assets based on pilot success and ROI.
MLOps (Machine Learning Operations)
Practices combining ML, DevOps, and data engineering to manage the ML lifecycle. This includes deploying, monitoring, managing, and governing models in production to ensure reliability and continuous improvement.
Real-World Results: Case Study Spotlight - US Water Utility
The Challenge: A US-based water utility faced high costs and service disruptions from unexpected equipment failures. Pumps, valves, and filters failed unpredictably. Although the utility had sensors monitoring pressure, temperature, quality, etc., their time-based maintenance didn’t effectively use this data, leading to reactive fixes instead of proactive ones.
The Solution: NeuroByte partnered with the utility to implement an AI-powered PdM strategy, unlocking the value in their existing sensor data.
- Assessment & Strategy: We identified critical assets based on failure impact and cost. Working with their maintenance teams, we pinpointed key failure modes (pump bearing wear, filter saturation, etc.) and looked for corresponding patterns in existing sensor data. The data audit assessed the quality and accessibility of data from installed sensors and operational systems (like SCADA - Supervisory Control and Data Acquisition).
- Data Infrastructure & Integration: We integrated disparate data streams, building pipelines to ingest real-time data from sensors, SCADA systems, and CMMS maintenance logs into a unified platform. We focused on data cleansing, validation, and alignment to ensure reliable model training data.
- AI Model Development: Using the integrated data, we developed machine learning models tailored to the utility’s equipment. We employed algorithms suited for time-series data and anomaly detection (like XGBoost, Isolation Forest, and LSTM networks) to identify patterns preceding failures, often weeks in advance.
- Deployment & Workflow Integration: We created a dashboard for the maintenance team showing equipment health scores, high failure probabilities, and predicted failure timelines (e.g., “Filter Station 3: 90% probability of needing media replacement in 10-12 days”). The dashboard highlighted key sensor readings driving predictions. We integrated high-priority alerts directly into their work order system, enabling condition-based scheduling.
- Change Management & Training: We conducted workshops to train staff on using the predictive insights. Demonstrating the system’s accuracy on pilot assets built trust and encouraged the shift from calendar-based to data-driven maintenance.
The Results: By using AI to leverage their existing sensor data, the utility achieved significant improvements:
- 20-30% Reduction in Overall Maintenance Costs: Minimized emergency repairs and optimized preventive action timing based on actual conditions, not fixed schedules.
- Improved Equipment Uptime & Service Reliability: Planned interventions during off-peak hours drastically reduced service disruptions.
- Extended Asset Lifespan: Early issue detection prevented major failures, prolonging infrastructure life.
- Optimized Resource Allocation: Maintenance focused on assets needing attention, improving labor efficiency and spare parts management.
- Enhanced Safety & Compliance: Proactive maintenance reduced operational risks and supported adherence to regulatory standards.
US Utility Case Study: AI-Powered PdM Flow
- Yes: Issue Proactive Maintenance Alerts & Update Dashboard.
- No: Maintain Routine Monitoring Schedule.
This case study shows how AI can unlock value from existing sensor investments, transforming maintenance from a reactive cost center to a proactive, strategic function.
Overcoming Hurdles: Navigating the Challenges
While PdM benefits are clear, implementation has challenges. Awareness and planning help:
Key Implementation Hurdles & Solutions
Data Quality/Availability: Perform data audit, start small, potentially add sensors.
Skills Gap: Partner with experts (like NeuroByte), train staff, use managed platforms.
Change Management: Involve teams early, show pilot value, provide training.
Initial Investment: Build strong ROI case, start with pilot, explore phased rollout.
Integration Complexity: Plan early, choose tools with good APIs, allocate resources.
Choosing the Right Partner & Your Next Steps
Successfully implementing AI-powered PdM often requires external expertise. Choosing the right partner is crucial. Look for:
- Industry Experience: Deep understanding of your specific sector and equipment.
- Proven AI/ML Expertise: Demonstrated success developing and deploying predictive models.
- End-to-End Capabilities: Ability to handle data assessment, infrastructure, modeling, integration, and support.
- Collaborative Approach: Willingness to work closely with your operations and maintenance teams.
- Focus on Business Value: Prioritizing solutions delivering tangible ROI aligned with your goals.
Ready to move from reactive to predictive?
Your Next Steps
Use this guide to evaluate your current maintenance practices and data readiness.
Pinpoint critical assets where PdM could deliver the biggest impact.
Schedule a complimentary Strategic Data & AI Workshop (link placeholder) with NeuroByte to discuss your specific challenges and explore tailored solutions.
Ready to Slash Maintenance Costs?
Discuss your specific predictive maintenance needs. Schedule a free, no-obligation discovery call today.
Schedule Free Discovery CallConclusion: The Future of Maintenance is Predictive
AI-powered predictive maintenance isn’t futuristic; it’s a practical, value-driven strategy available now. By leveraging your operational data, you can anticipate failures, cut maintenance costs, boost productivity, and gain a competitive edge.
Moving beyond reactive fixes requires a strategic shift in mindset, embracing data as a core asset for operational excellence and reliability.
While challenges exist, the substantial ROI and operational benefits make AI-powered PdM a compelling investment for forward-thinking leaders. Embrace the predictive advantage and transform your maintenance function into a powerhouse of efficiency and reliability.
Glossary of Terms
Computer systems designed to perform tasks that typically require human intelligence, such as learning, problem-solving, and decision-making.
A set of rules or instructions followed by a computer to solve a problem or perform a calculation (e.g., XGBoost, Isolation Forest, LSTM are specific types used in ML).
Identifying data points or events that deviate significantly from the expected pattern, often indicating a potential issue or failure.
A set of rules and protocols that allows different software applications to communicate and exchange data with each other.
Software used by organizations to manage maintenance activities, track work orders, and store asset information.
A systematic review of data sources to assess their availability, quality, format, and suitability for a specific purpose (like AI model training).
An automated process that moves data from its source (like sensors or databases) to a destination (like an analytics platform), often involving cleaning and transformation steps.
Business management software that integrates various functions like finance, HR, manufacturing, and supply chain management into one system.
The process of selecting, transforming, or creating relevant data inputs (features) from raw data to improve the performance of machine learning models.
Measurable values that demonstrate how effectively a company is achieving key business objectives.
A subset of AI where systems learn from data to identify patterns and make decisions or predictions without being explicitly programmed for each task.
Practices for collaboration and communication between data scientists and IT/operations professionals to help manage the production ML lifecycle (deploying, monitoring, and maintaining models).
A maintenance strategy that uses data analysis and ML techniques to predict potential equipment failures before they happen, allowing maintenance to be scheduled proactively.
A small-scale, initial implementation of a solution (like PdM on a few assets) to test feasibility, refine the approach, and demonstrate value before a full rollout.
A performance measure used to evaluate the efficiency or profitability of an investment. It compares the gain from an investment relative to its cost.
A system used in industrial settings to monitor and control equipment and processes remotely (common in utilities and manufacturing).
A sequence of data points collected over time (e.g., sensor readings taken every minute), often analyzed to identify trends, seasonality, or anomalies.